The Reinvention of BI Leadership in the AI Enterprise
At least weekly, I am granted the opportunity to meet and work alongside experienced professionals who serve in a corporate business intelligence (BI) leadership function. When they describe their role upon introduction, there is a common thread to the scope of influence and control which usually intersects one or more of these domains:
- “I oversee business requirements analysis and report or dashboard design”
- “I oversee the development of all managed and curated BI content”
- “I operate a center of excellence for specific BI toolsets, and/or corporate BI standards and methods”
- “I oversee management reporting only”
- “I oversee the data warehouse”
As the conversation progresses, we may talk about process, technology, achievements, failures and their roadmap, but inevitably we touch on what is changing in their world:
- “We rolled out and users are producing their own content now”
- “Few are asking for more reports – most just want more data these days”
- “We’ve reached a steady state for management reporting”
- “Usage is dropping on our core reporting platform – and those that do just pull data”
- “All the attention seems to be on AI and Machine Learning but I don’t know where to start”
It isn’t difficult to connect the dots between the observed symptoms of change and the scope of BI leadership before uneasiness and concern begins to set in. It’s not to say that anyone believes that the need for managed BI is going to disappear entirely. But rather, thanks to automation, democratized toolsets, and an increasingly tech savvy workforce, the resources required to deliver are on the decline and investments are moving to new areas.
Fortunately, most conversations I have are with BI leaders that demonstrate a passion for learning and adaptation, and an overwhelming desire to have a lasting impact on their organization. As the conversation turns more candid, we start to tackle some of the real burning questions among today’s BI leaders:
- “How can I best make a difference in my organization?”
- “Is it possible for me to contribute even if I am not a data scientist?”
- “How do I proactively manage my career and remain valuable to my organization and marketable to prospective employers?”
- “How do I do the same for the teams I’ve built and all the people that have trusted me and delivered for me in their current roles?”
The key to understanding how today’s BI leaders will help their organizations continue to progress their data-analytical maturity, and expand their scope of influence to contribute in new and meaningful ways, is honing in on what is newly painful, or increasingly valuable for their stakeholders, and building out new competency in those emerging problem domains. As BI tool democratization softens the single/central model for BI content development, and data science, machine learning and/or AI overtake BI as more powerful methods to support (and sometimes automate) business decision making, there are a number of new and old problems resurfacing that are in desperate need of attention.
Data Science & AI Leadership
The most enticing opportunity for BI leaders is to pivot into data science and AI leadership. With the support of an experienced data scientist, it IS POSSIBLE to capitalize on your strong organizational networks, deep cross-functional, institutional, and business process knowledge to become your organization’s AI leader and chief evangelist. You will most likely be learning as you go, which will require a certain degree of personal commitment including lots of self-study after hours, as well as strong partnerships to provide the necessary data-science backstop to be successful. If your C-suite or board has recently asked you, “what can we be doing with AI or machine learning?” you may want to seriously consider capitalizing on this opportunity to advance your career and progress your organization.
Data Governance
If your organization is all in with self-service analytics and data science as core operating principles, then chances are they have already recognized how their opportunities are limited until they alter business process to emphasize accurate measurement as much as efficient execution, implement new strategies for data collection, and help the organization’s analysts (now everyone in the self-service era) and data scientists to move faster by making all the data they use more easily understood, interpreted and integrated. While Data Governance is far from a new concept, we have found that data science and the proliferation of self-service have been a catalyst for new governance, master data management and data catalog initiatives. The latter data catalog concept is perfectly demonstrative of this new wave of data governance being focused on faster insights over control-alone. It is a nuanced but refreshing pivot that is easy for the organization to rally around.
Decision Support Experience Design
As organizations become more adept at doing data science and building more machine learning models, there is an often overlooked change management obstacle that stands between a functioning model, and recognizing the business benefits of AI and machine learning. Many, if not most, of the business processes that will benefit from machine learning are still human-in-the-loop, where a human operator is making decisions and taking actions based on the advice of our models.
We believe that design thinking is a powerful tool to apply in this context, and that we will see the rapid emergence of a discipline that is focused on designing user experiences (visual, business intelligence, chat, voice, augmented reality, automation, or otherwise) that surface predictions and prescriptive recommendations with clarity and transparency that is necessary to achieve the outcomes envisioned by the organization. We have dubbed this concept decision support experience design, but have also seen it referred to as integrated intelligence and/or augmented intelligence in other settings.
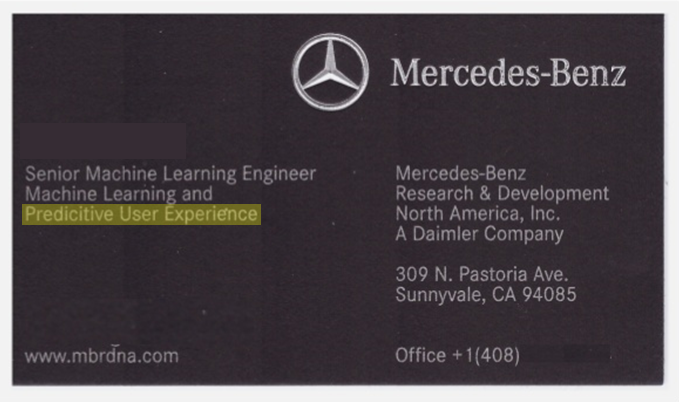
1 “Artificial Intelligence: How Enterprises Can Crush It With Apache Spark”
Business Process Optimization
It’s time to dust off that Six Sigma belt you earned in the 90s during your company’s massive ERP rollout. Savvy business leaders will recognize that AI is a powerful new capability in the pursuit of process optimization. Unlike pure BI, which is largely focused on reporting the news, AI business use cases are tightly intertwined with the business processes they seek to understand and improve and this is highlighting the need for a new flavor of governance leadership in the organization.
Take for example a machine learning model to predict customer churn, which would be used to optimize and automate customer retention business processes. For the organization to recognize the benefits of such an initiative, they would need to intimately understand existing business process, model where decisions are made, and leverage AI to optimize it with timely predictions. Today many organizations experimenting with AI are initially surprised by this relationship and often are resistant to implement process change, which in turn can stifle the anticipated benefits of any initiative.
We believe there are great benefits to be recognized by converging data science and process engineering teams within a business, and that there is a sustaining need for facilitators that possess extensive process and institutional business knowledge, as well as understand how AI can be wielded in order to fully recognize the business benefits. This role has a great deal of overlap with the aforementioned data governance role, which is one that will also be evangelizing process change, but more often in the context of advocating for better tracking, capture and measurement to make future AI-driven optimization possible.
Data Product Management
AI has been a catalyst for many organizations to begin producing data and analytical products for the direct or indirect benefit of stakeholders outside of the corporate walls. Consider the following data monetization examples below:
- Corporate franchisors are selling premium insights to franchisees to help them better operate their businesses
- B2B service providers like Cartus, once solely focused on process execution, are now using machine learning atop their mountains of historical data to power smart recommendations and improve customer experiences
- Retailers like Kroger and Dollar General are sharing retail sales data with suppliers to help them optimize merchandising and demand planning
If your organization is exploring how it can directly or indirectly monetize data and open up new markets, you may already have the domain knowledge and many of the necessary skills to assist or even lead such efforts.
Jump Start AI at your Organization
As you might have already concluded, most if not all of the above career paths have a great deal of overlap. Depending on the size and scale of your operation, it is possible that you may take on responsibilities across many of these new domains. If you’re a BI/DW leader in your organization and you are seeking to advance your organization or plan your next career move, Ironside will partner with you to deliver a private AI workshop customized to your business needs. Contact us today to learn more.
References
1 Gualtieri, Mark. “Artificial Intelligence: How Enterprises Can Crush It With Apache Spark” LinkedIn SlideShare, Forrester Research, 9 Feb. 2017, www.slideshare.net/SparkSummit/artificial-intelligence-how-enterprises-can-crush-it-with-apache-spark-keynote-by-mike-gualtieri
About Ironside
Ironside was founded in 1999 as an enterprise data and analytics solution provider and system integrator. Our clients hire us to acquire, enrich and measure their data so they can make smarter, better decisions about their business. No matter your industry or specific business challenges, Ironside has the experience, perspective and agility to help transform your analytic environment.