What’s keeping mid-market companies from leveraging advanced analytics and AI in 2023?
Midmarket companies use Advanced Analytics and AI to automate processes, glean strategic insights and make predictions at scale such as:
- Marketing – What is the next best offer for this client?
- Customer churn – Will this customer churn soon?
- Predictive maintenance – When will this machine or vehicle fail?
- Insurance- Will this person file a large claim?
- Healthcare – Will this person develop diabetes?
Companies can wait until their competitors, or new entrants leverage AI in their industry, or they can start the process now. There’s no doubt that the coming years will see AI applied to ever-increasing processes in the organization. The urgency is to start reaping the benefits before widespread adoption in your industry occurs.
The good news is that midmarket companies are still in the early stages of large-scale deployment of AI projects. A recent survey by Corinium Intelligence (Data Leadership: Top Cloud Analytics Mistakes – and How to Avoid Them) found that only 4% of respondents say their advanced analytics models and self-service tooling are fully scaled and integrated with business processes across the organization.
However, midmarket companies are actively scaling and experimenting with AI and Advanced Analytics in their business processes. The survey found that 53% are creating MVPs (Minimum Viable Products) and 36% are in the process of scaling advanced analytics and AI, well on their way to deployment.
AI adoption will transform business models over 2-5 years. The time to start is now.
What challenges do midmarket companies face as they define, build and deploy Advanced Analytics and AI technologies in their companies?
The Corinium Intelligence survey asked mid-market companies about the biggest mistakes they saw or experienced in deploying Advanced Analytics and AI. This survey of 100 data and analytics leaders from the financial services, insurance, telecoms, retail, and manufacturing sectors highlights the challenges enterprises face at each step of the data modernization journey – from designing the right data architecture to incorporating AI in business processes for competitive advantage.
59% of respondents cited inadequate data and compute infrastructure as the leading impediment. Choosing the right technologies, hiring the right skill sets and proactively investing in change management are the next three sources of mistakes on the path to utilizing the AI/Advanced Analytics.
Choosing the wrong analytics or AI technology solutions can result in setbacks later on. It’s important to carefully consider the various analytics and AI solutions that are available and choose the one that best meets the needs of the organization.
Successful analytics and AI projects require a range of technical and domain-specific skills. 54% of survey respondents said it was important that the necessary skills and expertise are available within the organization, or that they can be acquired through training, hiring and partnering. In fact, many mid-tier companies bring in external expertise to implement AI and advanced analytics.
Almost half of the respondents identified failure to invest in change management as another risk. Analytics and AI projects can involve significant changes to processes. It’s important to proactively identify cultural and organizational success factors. This includes getting executive buy-in, aligning analytics and AI strategy with business goals and communicating the value of analytics and AI projects to the rest of the organization, in order to build support and ensure successful adoption.
The stakes are high. The mistakes leaders cited led to significant or total disruption of Advanced Analytics and AI strategies. These challenges can delay realizing the business benefits, delay advantages against competitors or hamper defending against new entrants who use Advanced Analytics and AI.
What are the options when building a world class advanced analytics and AI capability in my organization?
Three paths that companies follow include:
1. Build the capability in-house
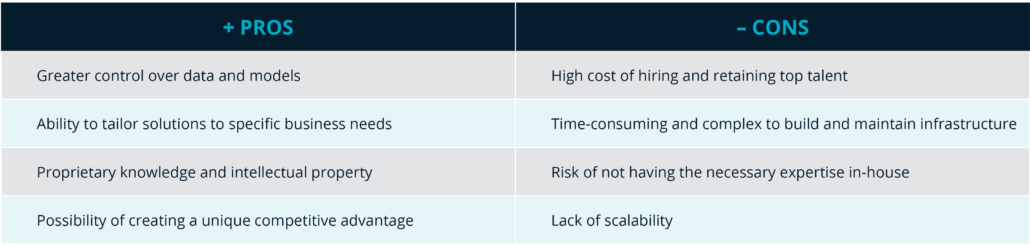
2. Buy third-party solutions
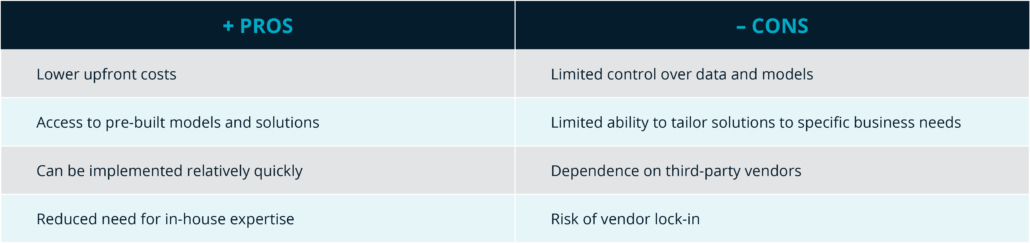
3. Partner with cloud consultants to accelerate customized advanced analytics/AI solutions
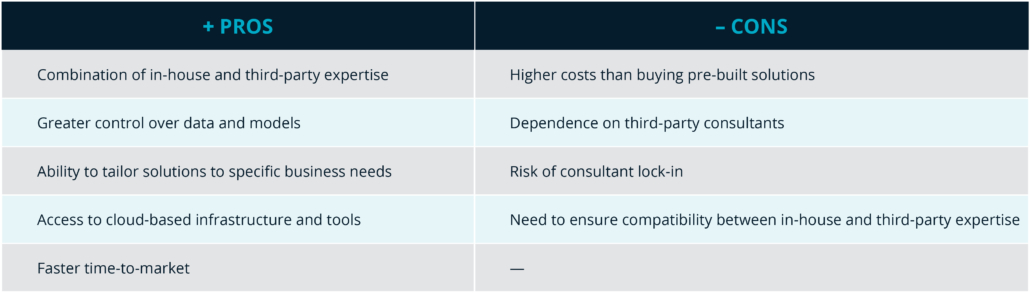
In summary, building Advanced Analytics/AI in-house offers greater control and the ability to tailor solutions to specific business needs, but it can be costly and time-consuming. Buying third-party solutions is quicker and less expensive, but it offers less control and limited ability to tailor solutions. Partnering with a cloud consultant can be a good middle ground as it provides a combination of in-house and third-party expertise and the ability to tailor solutions to specific business needs, but it is more expensive than buying pre-built solutions. Whichever path you choose, the benefits of advanced analytics and AI are well within your reach.
Take a look at the full whitepaper to learn more: Data Leadership: Top Cloud Analytics Mistakes – and How to Avoid Them
Contact Ironside Group today to accelerate your Advanced Analytics and AI Strategies.