When it comes to AI and automated machine learning, more data is good — location data is even better.
At Data Con LA 2019, I had the pleasure of co-presenting a tutorial session with Pitney Bowes Technical Director Dan Kernaghan. We told an audience of data analysts and budding data scientists about the evolution of location data for big data and how location intelligence can add significant and new value to a wide range of data science and machine learning business use cases.
Speeding model runs by using pre-processed data
What Pitney Bowes has done is take care of the heavy lifting of processing GIS-based data so that comes ready to be used with machine learning algorithms. Through a process called reverse geocoding, locations expressed as latitude/longitude are converted to addresses, dramatically reducing the time it takes to prepare the data for analysis.
With this approach, each address is then associated with a unique and persistent identifier, the pbKey™, and put into a plain text file along with 9,100 attributes associated with that address. Depending on your use case, then, you can enrich your analysis with subsets of this information, such as crime data, fire or flood risk, building details, mortgage information, and demographics like median household income, age or purchasing power.
Surfacing predictors of summer rental demand: location-based attributes
For Data Con LA, we designed a use case that we could enrich with location data: a machine learning model to predict summer revenue for a fictional rental property in Boston. We started with “first person” data on 1,070 rental listings in greater Boston that we sourced from an online property booking service. That data included attributes about the properties themselves (type, number of bathrooms/bedrooms, text description, etc.), the hosts, and summer booking history.
Then we layered in location data from Pitney Bowes for each rental property, based on its address: distance to nearest public transit, geodemographics (CAMEO), financial stress of city block, population of city block, and the like.
Not surprisingly, the previous year’s summer booking and scores based on the description ranked as the most important features of a property. However, it was unexpected that distance to the nearest airport ranked third in importance. Other location-based features that surfaced as important predictors of summer demand included distance to Amtrak stations, highway exits and MBTA stations; block population and density measures; and block socio-economic measures.
By adding location data to our model, we increased the accuracy of our prediction of how frequently “our” property would be rented. Predicting that future is an important outcome, but more important is determining what we can do to change future results. In this scenario, we can change the price, for example, and rerun the model until we find the combination of price and number of days rented that we need to meet our revenue objective.
Building effective use cases for data science
As a Business Partner since 2015, Ironside Group often incorporates Pitney Bowes data — both pbKey flat-file data and traditional GIS-based datasets like geofences — into customized data science solutions built to help companies grow revenue, maximize efficiency, or understand and minimize risk. Here are some examples of use cases that incorporate some element of location-based data into the model design.
Retail loss prevention. A retailer wanting to analyze shortages, cash loss and safety risks expected that store location would be a strong predictor of losses or credit card fraud. However, models using historical store data and third-party crime risk data found that crime in the area was not a predictor of losses. Instead, the degree of manager training in loss prevention was the most significant predictor — a finding that influenced both store location decisions and investments in employee training programs.
Predictive policing. A city police department wanted to a data-driven, data science-based approach to complementing its fledgling “hot spot” policing system. The solution leverages historical crime incident data combined with weather data to produce an accurate crime forecast for each patrol shift. Patrol officers are deployed in real time to “hot spots” via a map-based mobile app. Over a 20-week study, the department saw a 43% reduction in targeted crime types.
Maximize efficiencies for utilities demand forecasting. A large natural gas and electricity utilities provider needed a better way to anticipate demand in different areas of their network to avoid supply problems and service gaps. The predictive analytics platform developed for the utility uses cleaned and transformed first-party data from over 40 different geographic points of delivery, enriched with geographic and weather data to improve the model’s predictions of demand. The result is a forecasting platform that triggers alerts automatically and allows proactive energy supply adjustments based on predictive trends.
About Ironside Group and Pitney Bowes
Ironside Group was founded in 1999 as an enterprise data and analytics solution provider and system integrator. Our data science practice is built on helping clients to organize, enrich, report and predict outcomes with data. Our partnership and collaboration with Pitney Bowes lead to client successes as we combine our use case-based approach to data science with Pitney Bowes data sets and tools.
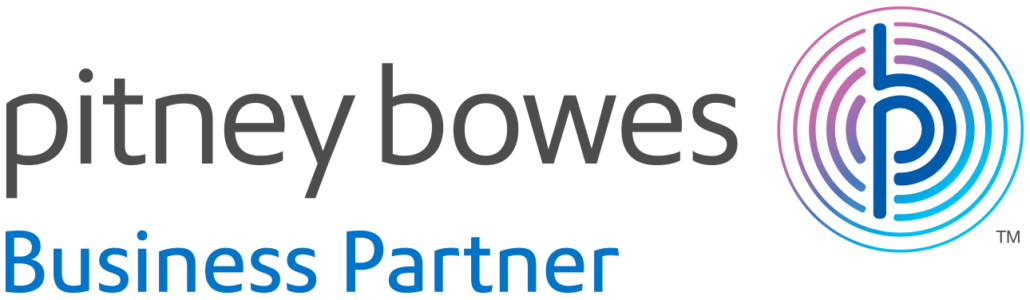