What causes advanced analytics and AI initiatives to fail? Some of the main reasons include not having the right compute infrastructure, not having a foundation of trusted data, choosing the wrong solution or technology for the task at hand and lacking staff with the right skill sets. Many organizations deploy minimum valuable products (MVP) but fail to successfully scale them across their business. The solution? Outsourcing elements of analytics and AI strategy in order to ensure success and gain true value.
64% of leaders surveyed said they lacked the in-house capabilities to support data-and-analytics initiatives.
It’s essential to implement a data-driven culture across your organization if you’re looking to adopt advanced analytics. One of the keys to a data-driven culture is having staff with the correct skills that align with your initiatives. In our study, 64 out of 100 leaders identified a lack of staff with the right skills as a barrier to adopting advanced analytics within their organization. Even for organizations that do have the correct skill sets, retaining that talent is also a barrier they face. This is where outsourcing comes in.
Borrowing the right talent for only as long as you need it can be an efficient path forward.
Outsourcing parts of your analytics journey means you’re going directly to the experts in the field. Instead of spending time and money searching for the right person both technically and culturally, outsourcing allows you to “borrow” that talent. The company you choose to outsource to has already vetted their employees and done the heavy lifting for you. With outsourcing, you can trust that your organization is working with professionals with the skill sets you need.
Aside from securing professionals with the correct skill sets, there are plenty of other benefits to outsourcing your organization’s analytics needs. Professionals with the skill sets necessary for advanced analytics and AI initiatives can be very expensive. Outsourcing provides a cost-effective option to achieve the same goal. Rather than paying the full-time salary and benefits of a data science or analytics professional, an organization can test the value of these kinds of ventures on a project to project basis and then evaluate the need for a long-term investment.
Freeing full-time employees to make the most of their institutional knowledge.
Another benefit of outsourcing analytics is the increased productivity and focus of your organization’s full-time employees. By outsourcing your organization’s analytics, your full-time employees will naturally have more bandwidth to focus on other high priority tasks and initiatives. Rather than spending their time on what the outsourcing company is now working on, the full-time employees can dedicate their time to work on things that may require institutional knowledge or other tasks that are not suited for a third party. It’s a win-win situation for your organization – your analytics needs are being handled and your full-time staff is more focused and still productive.
There are many areas of analytics that an organization can outsource. These areas include but are not limited to viability assessments, prioritization of use cases, managing the ongoing monitoring, performance, maintenance and governance of a solution and implementing and deploying an MVP or use case. In the words of Brian Platt, Ironside’s Practice Director of Data Science, “A partner with advanced analytics and data science capabilities can rapidly address AI challenges with skills and experience that are hard to develop in-house.”
Mid-tier organizations need the right talent and tools to successfully realize the value of their data and analytics framework in the cloud. The Corinium report shows that many companies are increasingly prepared to work with cloud consulting partners to access the skills and capabilities they require.
Areas that mid-market leaders consider outsourcing.
Overall, more and more data leaders are turning to outsourcing to help fill the gaps and expedite their organization’s analytics journey. Outsourcing services can help your organization reach analytics goals in many different areas, not just AI and Advanced Analytics.
Organizations rely on outsourcing in key areas like these:
- Developing a data and analytics cloud roadmap
- Assessing advanced analytics use cases (figure shows 68% would consider outsourcing)
- Implementation and deployment of a MVP, or use case (figure shows 43% outsource)
- Developing and maintaining data pipelines
- Documenting and assessing your BI and overall analytics environment(s)
- Migrating your reporting environment from one technology to another
- Overall management and monitoring of analytics or AI platform (figure shows 42% are already outsourcing)
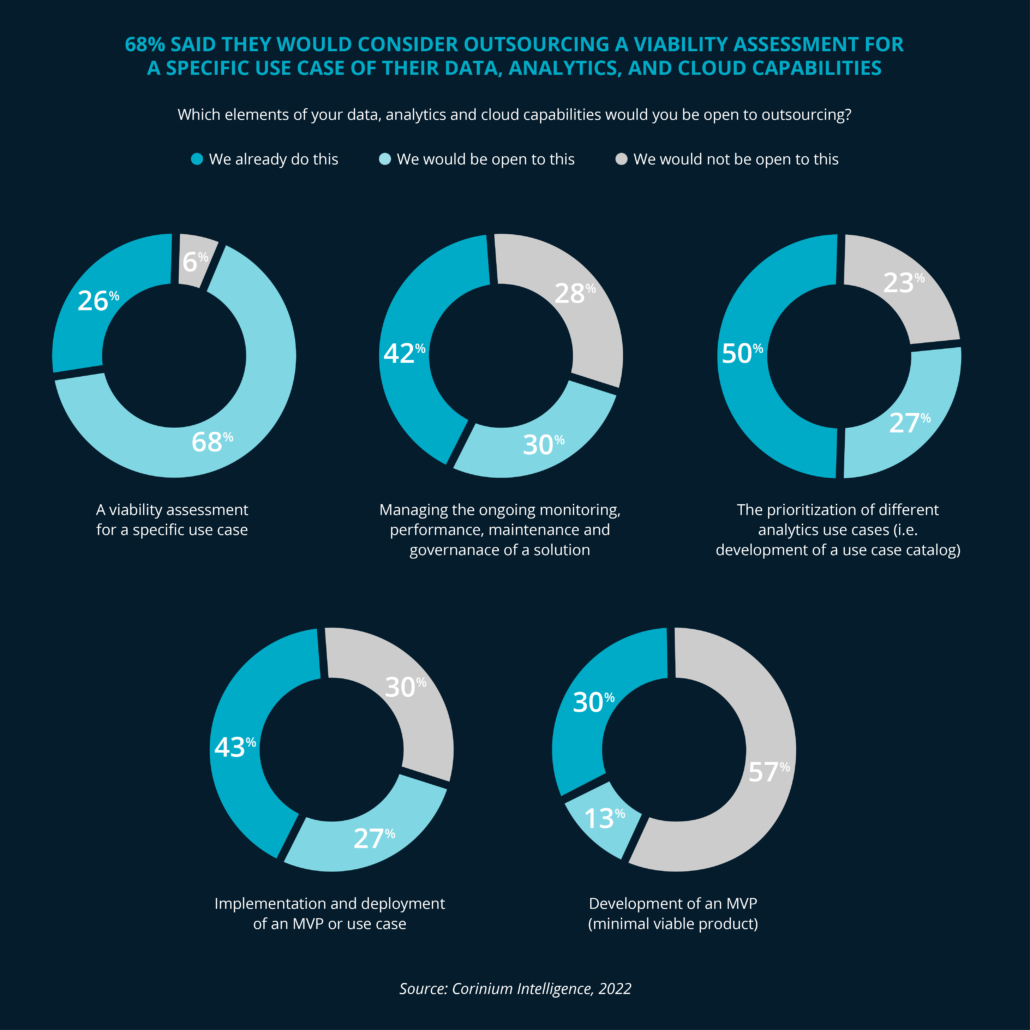
When your company plugs into the right skill sets and processes, there’s nothing between you and a successful data-and-analytics transformation.
Take a look at the full whitepaper to learn more: Data Leadership: Top Cloud Analytics Mistakes – and How to Avoid Them
Contact Ironside Group today to accelerate your Advanced Analytics and AI Strategies.